How Machine Learning Can Transform Your Demand Planning
Brian Hoey - April 04, 2019
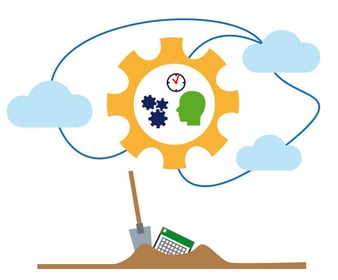
Let’s talk for a second about pattern recognition. The human brain is constantly searching the perceptible world for patterns, sometimes in order to make better decisions (in the case of, say, emerging traffic patterns while driving) and sometimes simply in order to pass the time (in the case of constellations). The thing is, while the search for patterns is an innately human pastime, it’s not something that we as a species are necessarily all that good at. Think about it: how often do we read or hear about people making the same mistakes over and over again without identifying the common factor? How often do we see businesses rolling out the same strategies over and over again without ever noticing the ways in which those strategies could be improved?
This is as true in supply chain management as it is anywhere else, and it’s not hard to imagine why. More so than many other industries or business functions, SCM involves a number of moving parts and disparate information sources that’s usually too great for a single human planner to grapple with effectively. What’s the solution here? Possibly, it’s implementing technology explicitly designed around more effective pattern recognition. That’s right, we’re talking about machine learning.
Reducing Forecast Error
Machine learning operates on the principle that, given the right feedback, an algorithm can learn to uncover hidden links in large, complex datasets. These are typically patterns that would be virtually impossible for a human planner to uncover. When applied to demand planning in particular, this might lead to a situation in which your business runs an ML algorithm on real-time market data, combined into one data lake with the operational info you’ve been collecting on your production processes and other supply chain management figures, in order to estimate in advance the likely demand levels for a new product. Conversely, the same process could be used to estimate likely changes in demand levels before they happen, giving your organization enough time to optimize production ratios and inventory levels accordingly.
Again, these forecasts will be based on connections that a human planner couldn’t realistically be expected to see with the naked eye. The immediate result is improved demand planning by way of a smaller gap between expected demand and real demand, i.e. you face fewer areas of disconnect between expectations and reality, empowering you to plan your production, warehousing, and transport flows in a more optimal manner. In this way, you can avoid the effects of poor demand forecasting: costly storage of unsold goods, late product deliveries due to stock shortages, inefficient bundling of freight, etc. More than all that, however, you put yourself in a position to make more substantial changes to the way that you manage demand throughout your various product lifecycles. The questions is: what are these changes, and how does ML help make them a reality?
The Joys of Visibility
In the scenarios we’ve been laying out above, your machine learning processes have been gathering data from numerous sources across the supply chain. In an ideal world, that data is being gathered in real-time, in order to create the most agile and responsive possible predictive processes. But it’s not just the ability to take in data and spit out predictions that has value for your demand planning processes. On the contrary, the fact that these data streams are all being centralized means that your overall operational visibility should improve, independent of the actual predictions that are being produced. This means that, as a planner, you’re also putting yourself in a position where you can access individual pieces of information with relative ease. Likewise, your colleagues spread across different touchpoints on the value chain can also gain insight into your processes as they unfold. As a result, your entire organization (to say nothing of partners up and downstream) can tackle demand planning in a more open, collaborative way.
What does this mean in practice? Well, imagine that you’re sourcing raw materials for a few different production workflows. Your advanced analytics give you some estimates into the likely demand levels for various products in the coming quarter, but they also connect you with data from your various suppliers. Thus, you can weigh the costs of meeting demand with the availabilities of various parts. Is it more economical to place a large order now so that you can meet demand later without having to worry about potential sourcing issues down the road, or should you work with your supplier to figure out rates and purchase orders that create synergy between both organizations? This is a level of planning optimization that goes beyond simply arranging your value chain activities around more accurate forecasts—it represents a smarter way of rolling out your production plans, keeping the supply chain in its entirety within view at all times.
Demand Capacity Optimization
Now, once you’ve gotten a firmer handle on demand, it’s time to think about the other side of the curve: capacity. After all, knowing in advance that there will be a spike in demand only helps you if you have the capacity (to say nothing of the flexibility) to actually meet that demand. Here, again, machine learning presents a potential path forward. How? By powering the kinds of prescriptive analytics workflows that go hand in hand with the predictive ones we discussed above. Using these types of processes, it’s possible to discover not just an accurate picture of your maximum production or shipping capacity, but potential ways to improve that capacity.
This can take many forms, but one common use case involves digitally modeling your entire factory floor (potentially utilizing IoT data to create real-time information streams) and letting your analytics workflows test various alternative scenarios for, say, machine arrangement. In this way, you can find smarter, more efficient ways to structure your operations in order to maximize your capacity levels. Thus, you can put yourself in a position to accommodate higher demand levels if and when they arise. After all, you’ll have plenty of advanced notice when that demand change occurs—thanks to the changes that machine learning has brought to your demand planning workflows.
LATEST POSTS
- Understand Circular Economy in The Manufacturing Industry
- How Can Industry 4.0 IT Integration Be Achieved Smoothly?
- The Significance of Order Sequencing in Discrete Manufacturing
- How to improve your Supply Chain Management: The Power of Control Towers
- Optimizing Human Resource Scheduling in Manufacturing: A Technological Approach