5 Uses for Machine Learning in Supply Chain Management
Brian Hoey - August 16, 2018
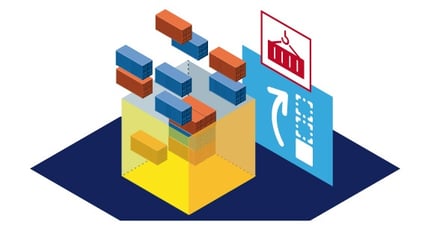
New technologies—from steam power to modern computers—have been the driving forces in supply chain management since the beginnings of industrialized society. Today, supply chain technology is changing at an exponential rate, providing supply chain planners with possibilities that would have seemed like science fiction even a few decades ago. If you follow the news and trends in SCM, you’ve no doubt noticed that machine learning (ML) is often touted as the next major innovation in this long line of technological evolutions—but what, exactly, is it, and how can supply chain managers put it to use?
1. Scheduling Maintenance
Simply put, machine learning is a process that involves examining quantities of data so large that it would be impossible or impractical for a human to comprehend them and gain meaningful insights. Today, ML is the technology that makes e-mail spam filtering possible, as well as a host of other processes that have become fairly commonplace. In the supply chain, however, its untapped power is just beginning to make itself apparent. One of the most exciting early applications of this technology in an industrial setting is proactive machine maintenance scheduling, in which advanced ML algorithms study the signs of machine failure and predict in advance which pieces of machinery are likely to experience a breakdown or malfunction in the near future. This way, planners can schedule downtime in advance, before a breakdown occurs, in such a way as to be minimally disruptive to other processes. While workflows like this are theoretically possible in less technologically advanced settings, the advanced algorithms at work in these situations can predict potential malfunctions with a degree of accuracy unthinkable for human planner. Trucks and other elements of the supply chain can, by the same token, also benefit from this application.
2. Identifying Product Damage
Another emerging application of machine learning in the supply chain is using machine sensors to identify damaged products. This can take numerous forms, from heat and light sensors in trucks and containers that can determine if cargo has become overheated to computer vision technology that scans inbound goods for damage during inventory stocking. In both of these examples, the need to manually check items as they’re taken in or unloaded is greatly reduced, and, in the latter example, the speed with which items are check is significantly increased (owing to the algorithm’s ability to comprehend visual data much more quickly than a human could). Not only can damage be identified, Industry 4.0-enabled systems can automatically send out notifications and make simply adjustments once the faulty parts are discovered, thereby helping to minimize disruption across all points on the value stream.
3. Improving Demand Forecasting
It’s possible that this section should be split up into two separate use cases, but these two paths that ML workflows can take still fundamentally lead to the same place: smarter, more accurate demand forecasting. On the one hand, by analyzing huge quantities of data, ML algorithms can improve the quality of advanced analytics processes like forecast generation and other predictions. Conversely, it’s also possible to use machine learning to do in-depth analyses of individual customers or clients in order to predict future buying and ordering behavior. In this way, businesses are not just able to better tailor their production and transport processes to real demand, they’re also able to improve their partnerships with particular customers. By anticipating and acting on the particular needs of your customers before they arise, your business can establish itself as a trusted partner capable of adding significant value above and beyond the value of your products.
4. Streamlining Production Planning
Above, we alluded to the possibility of production streams being alerted when damaged goods are identified and need to be replaced. When it comes to ML’s role in optimizing complex production plans, this is only the tip of the iceberg. In point of fact, sophisticated algorithms can be trained on your existing production data in such a way as to identify possible areas of waste and inefficiency, as well as to create a more adaptable environment in which any given disruption is met with a suitable plan b. While these sorts of benefits would no doubt be welcome in any production plant, they can be particularly impactful in non-clocked or job shop production environments, owing to the inherent difficult of finding the optimal job shop schedule by hand.
5. Boosting End-to-End Visibility
Visibility has remained something of a buzzword in supply chain circles for the past several years or longer, and each new technology that crops up promises to improve visibility in some meaningful way. So what will machine learning’s contribution to this trend be? Essentially, these complex algorithms are giving some supply chain planners new opportunities for gaining a sense of order and cohesion in an increasingly disparate, complex, and changeable global value chain. By examining data from a host of different sources, ML workflows are able to discover complex interconnections between various disparate processes along far-flung points on the value chain. To wit, the success of any given ML process should—as with any new technology—be defined by how well it enables users to cut through complexity. After all, cutting through complexity is already at the heart of successful supply chain operations.
LATEST POSTS
- Understand Why Production Planning Needs Specialized Solutions
- Understand Circular Economy in The Manufacturing Industry
- How Can Industry 4.0 IT Integration Be Achieved Smoothly?
- The Significance of Order Sequencing in Discrete Manufacturing
- How to improve your Supply Chain Management: The Power of Control Towers