How Machine Learning Impacts Supply Chain Management
Nick Ostdick - September 07, 2017
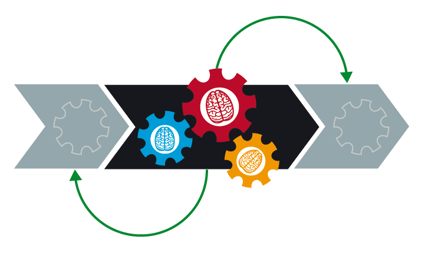
The manufacturing industry has long relied and functioned on a platform of automation or an automated method of facilitating production programs. The production facility floor is ground zero for how manufacturing companies use robotics or artificial intelligence to streamline production processes and increase the efficiency of how products move from the production floor to the customer’s door. But what’s slowly gaining more and more prominence in the manufacturing industry is machine learning outside of the actual production space and the ways in which a digitized manufacturing platform can enhance supply chain management at every touch point of the value stream.
Understanding machine learning in this context — a holistic reimagination of how this technology can be a disruptive force in a cross-organizational way from sales and procurement to transport logistics — puts machine learning on a grander stage in terms of shaping the future of the automotive supply chain. In addition, machine learning can provide planners and managers with a critical competitive advantage in a somewhat uncertain, variant-rich manufacturing space.
With the help of technologies and principles such as Industry 4.0, Big Data, and advanced analytics, let’s examine a handful of ways in which machine learning impacts supply chain management and what planners and managers can learn from deploying machine learning in such a way.
Condition monitoring and management
Much has been discussed revolving machine learning and its ability to help manufacturing companies produce better quality parts or products with longer lifespans. Because machine learning deploys a greater degree of accuracy during planned production programs, the end product can be of higher quality and greater complexity, which is critical as the manufacturing landscape and its associated technology continues to advance and evolve. But machine learning also offers the ability for planners and managers to monitor the condition of parts at various stages in production to detect potential faults, failures, or defects during production in real-time and take the necessary steps to correct these errors. This reduces costs associated with large batches of faulty parts or large-scale recalls if defects are detected after parts leave the production line or warehouse. Condition monitoring also helps planners and managers conduct detailed forecasting and what-if scenarios to help combat future breakdowns or bottlenecks in production programs.
Quality control and process enhancement
The future of quality control in the automotive supply chain is likely to change drastically in the face of machine learning. Gone are the days when products are tested or evaluated in the prototype setting or even following small production runs. Instead, machine learning — in conjunction with Big Data and other powerful information gathering and reporting strategies — will allow planners and managers to predict quality early in the production process and make necessary adjustments to ensure the right level of quality for the right product. Machine learning will help planners and managers gather data on the shifting conditions or transformations of materials in order to detect potential defects. This information can then be communicated to those across various touch points of the supply chain to help avoid shortages, stoppages, or other disruptions detrimental to servicing clients and customers.
Energy efficiency and conservation
One of the bigger conversations in the automotive supply chain during the last year was the continued development of green technology and the push for increasingly energy efficient production and transportation models. The move toward more eco-friendly manufacturing platforms not only benefits the environment, but it also allows companies to deploy new, innovative, and often cost-saving methods of production, warehousing, and transportation. Machine learning can play a large part in continuing this trend towards a more green supply chain by helping to predict fluctuations in future demand, which can help planners and managers best allocate and schedule resources and jobs. Machine learning also allows for this to be completed in real-time to help create the ultimate in end-to-end (E2E) visibility in terms of how companies are using energy and if energy usage could be directed to other production programs or facilities for more optimal results.
The real value of machine learning?
At the end of the day, the name of the game in the automotive supply chain is efficiency and transparency. Production programs need to be efficient, but planners and managers also need a window into the people and processes that make production happen on large and small scales. This is where machine learning not only matters greatly to how manufacturers will operate in the mid and long-term future, but will also have a significant impact on other associated industries as well, particularly in the tech sector, which is becoming more and more entwined with the automotive industry. Machine learning matters because as the automotive industry continues to diversify and coordinates with other industries to produce vehicles for an increasingly varying customer base, the alignment behind intelligent manufacturing principles will be the baseline for effective operations.
LATEST POSTS
- Understand Circular Economy in The Manufacturing Industry
- How Can Industry 4.0 IT Integration Be Achieved Smoothly?
- The Significance of Order Sequencing in Discrete Manufacturing
- How to improve your Supply Chain Management: The Power of Control Towers
- Optimizing Human Resource Scheduling in Manufacturing: A Technological Approach