Machine Learning vs. Intelligent Manufacturing
Brian Hoey - March 13, 2018
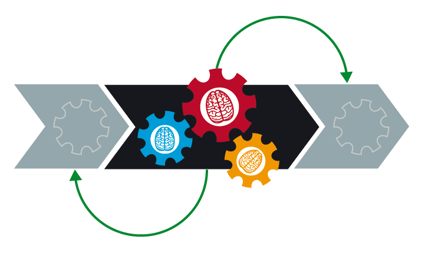
Blockchain. Big Data. Industry 4.0. Smart Factories. Terms like these get thrown around with some regularity whenever the future of manufacturing is under discussion. Often, it’s difficult to cut through the jargon and get to the matter that’s most relevant to you, i.e. what is this new technology and how will it impact your business? It’s a strange new world in modern manufacturing, and we at the flexis blog are committed to demystifying the terms that you may be hearing more and more frequently as you evaluate the ongoing health of your supply chain. To that end, let’s talk about machine learning, intelligent manufacturing, and the difference between the two.
What is machine learning?
Simply put, machine learning is a process in computer science whereby algorithms are trained on extremely large datasets in order to find relationships between different factors that would be otherwise impossible for humans to discern. When news outlets and consulting firms hype the power of Big Data, they often refer to it within the context of machine learning (though in some circumstances they are talking about data mining, a related process). Machine learning enables businesses or other entities with high data visibility to create complex mathematical models that can be used for more accurate predictions and prescriptive insights.
Machine learning processes are at work all around us, from optical character recognition (OCR) to e-mail spam filtering, but they can be especially impactful in variant rich industries like automotive manufacturing. Just as, on a small scale, an e-mail filter can learn to detect the individual factors that correlate most strongly with an e-mail being spam, machine learning algorithms trained on larger scale production processes can uncover factors associated with waste or inefficiency. By developing a highly nuanced model of the complex relationships between all touch points on the value chain, these algorithms can track the impact of seemingly unrelated workflows on one another and help planners to optimize various production processes in ways that would have been impossible without machine intervention.
What is intelligent manufacturing?
Intelligent manufacturing, on the other hand, overlaps significantly with the use of Industry 4.0 principles like interoperability and end-to-end (E2E) visibility. By prioritizing computer controls, connectivity across devices and services, and purposeful data collection, intelligent manufacturing helps to promote more agile, adaptable supply chains. Rapid prototyping, virtual factory interfaces, and real-time or machine-to-machine communication all combine to create to a smart factory environment that promotes new efficiencies and workflow improvements.
As is the case with Industry 4.0 itself, intelligent manufacturing can act as a significant value added proposition. Many manufacturers struggle to find the optimal balance when it comes to scheduling production, managing inventory, and tailoring throughput to shifting demand requirements and shipping windows. Making matters more complicated is the fact that each of these processes can potentially impact the others, meaning that decisions made in a siloized manner with low intra-operational visibility can have far-reaching and unintended consequences. Even businesses with a “plan for every part” approach will discover slowdowns and bottlenecks that can have significant ramifications for their operations. Intelligent manufacturing makes it possible to mitigate these potential bottlenecks with more transparent planning and logistics workflows.
A match made in heaven
By now it should be no surprise that machine learning and intelligent manufacturing, while distinct concepts within Industry 4.0, can and should go hand in hand when it comes to driving improvements in overall efficiency across business operations. More specifically, machine learning can be a major part of intelligent manufacturing, bringing an added level of predictive power and holistic insight to the process of eliminating waste and creating a leaner value chain. By integrating these two functions, manufacturers can add value and ultimately increase both revenue and customer satisfaction in a number of ways:
- Improved forecasts based on predictive analytics can help bring production plans more in line with real demand, which can be monitored and adjusted for in real-time.
- Machine learning algorithms can be trained on internet-of-things (IoT) sensors to predict wear and tear on factory floor machinery; this enables planners to schedule maintenance downtime in advance, both avoiding breakdowns and increasing supply chain stability and on-time delivery of goods.
- Prescriptive supply chain analytics can suggest workflow improvements that in turn improve data quality, boosting planning-side visibility.
- Some small, day-to-day decisions like routing adjustments and inventory restocking can be taken over by machines, freeing up valuable human planning-hours for more complex and pertinent tasks.
Truly, machine learning and intelligent manufacturing are a match made in heaven, containing the possibility of a manufacturing processes defined by agility and adaptability. GE estimates that proactive maintenance scheduling alone can help businesses recover up to 20% of their production capacity. For manufacturers who can adopt these new technologies and workflows, they can come to represent a significant competitive advantage over businesses that are either not successfully gathering data or failing to get the most from it.
LATEST POSTS
- Understand Circular Economy in The Manufacturing Industry
- How Can Industry 4.0 IT Integration Be Achieved Smoothly?
- The Significance of Order Sequencing in Discrete Manufacturing
- How to improve your Supply Chain Management: The Power of Control Towers
- Optimizing Human Resource Scheduling in Manufacturing: A Technological Approach