5 Steps for Smooth Advanced Analytics Implementation
Brian Hoey - May 07, 2020
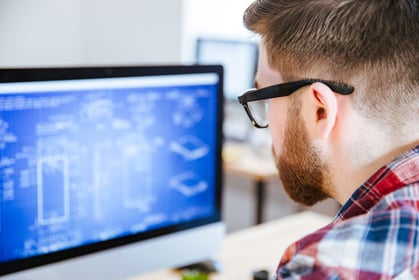
When you release a new product or service offering, what has to happen to get the project off the ground? If the new product initiative is coming from the C-suite, there’s a good chance that you’ll need to flesh out the original proposal and get buy-in from the technical personnel who will actually be designing and producing the new offering. You’ll need a roadmap that covers your goals and your path to turning a profit, plus a short term account of how best to utilize your various corporate resources during the ramp-up period. Without these things in place, your new offering isn’t terribly likely to be a success.
When it comes to a big change within your organization—say, advanced analytics implementation—you’re in a similar situation. The same level of care and planning needs to be poured into the process from the outset, otherwise you risk a poor ROI for your efforts. What does treating analytics implementation this way actually look like in practice? We’re glad you asked!
1. Identify Top Priorities for Advanced Analytics Implementation
It’s a frequently-cited myth that companies need to overhaul everything they’re doing all at once in order to make advanced analytics a key value-driver for their operations—but in reality that’s not necessarily the case. Especially for larger businesses that might have any number of large caches of unstructured or un-sanitized data, it can make sense to take a more targeted approach. An automotive manufacturer, for instance, might start out by limiting the scope of their deployment to factory floor data, leaving out inventory and logistics, in order to set themselves up with a manageable task. This can then serve as a proof of concept for further deployments, but you should make sure the scope is large enough to have a real ROI potential. From there, you can begin to map out all of the necessary IT tasks that will go into the implementation, begin to figure out your initial KPIs, and sketch out your potential resource usage for the project.
2. Identify Quick Wins
As you establish your priorities, you’ll also want to establish some goals, e.g. the KPIs where you expect to see significant operational improvement as a result of your new analytics processes. Again, for a manufacturer this might be a reduction in machine outages, with the ultimate goal of extending the functional lives of those machines, thereby saving money in the long run. This kind of long-term thinking is mission critical—but it needs to be complemented by some quick wins. The success or failure of this project will depend in large part on your ability to get stakeholder buy-in across your organization. What’s the best way to do that? By highlighting business cases were analytics will save you money immediately. In an industrial setting, advanced prescriptive analytics might be your best bet for this: With production and machine data being fed into your analytics engine, you can create a Digital Twin of your factory floor in order to run simulations for different production plans, machine arrangements, etc. From there, you can identify and eliminate any areas of waste or inefficiency within your production network.
3. Choose the Right Vendor
Some businesses will have, or at least be able to scale up to, full-blown in-house data analysis capabilities when they get started on an initiative like this. If that's not your situation, however, you’ll probably have to find a vendor who can provide an analytics platform and support for said platform. Why isn’t a step that’s this crucial earlier on the list? Simple: you don’t know what to look for in a vendor until you’ve got a roadmap and some quick wins already mapped out. Depending on your business and your operational priorities, you might be in the market for a solution that can easily integrate with different IT and take in data from disparate sources across the value chain. You might have preferences for cloud-hosted versus on-premise. You might have particular UI or accessibility requirements that will make or break your deployment. This is another instance where it’s critical to get buy-in from across your operation—this way you can make sure that everyone's priorities are aligned, and you can narrow down your vendors more confidently.
4. Bridge the Gap Between Analytics and Planning
One of the biggest pitfalls in advanced analytics implementation is the inadvertent creation of silos. All too often, you see organizations with robust analytics capabilities that fail to improve their production accuracy, their overall downtime, or their on-time delivery rates—all because the insights being developed in the analytics department aren’t making their way to the planners that would benefit from them. Far from being an afterthought once your deployment is off the ground, this is an issue that should be a concern from the very moment you start implementing advanced analytics in your supply chain. In order to make sure that silos don’t crop up around your analytics insights, it might be helpful to implement S&OP (sales and operations planning) and S&OE (sales and operations execution) meetings that are explicitly designed around analytics-powered forecasting. If you already have meetings like these in place, you’ll want to figure out as early as possible how you’re going to incorporate your newly-discovered insights into your existing processes.
5. Collect More Data
Last but not least: the success of your analytics integration will depend in large part on your ability to provide high quality data to whatever system you’re using. If you’re a manufacturer or a logistics provider, you probably generate tremendous amounts of data every day—but not all data is created equal. Going forward, you’ll want to do your best to make sure that a) the data you’re collecting is being sanitized and stored in a way that maximizes its value to your analytics team, b) to continue adding new sources of data where appropriate. This might mean linking your supply chain software to that of your suppliers, in order to get end-to-end insights into your value stream. This could even mean collecting more data from your customers (with their consent) in order to get an even more comprehensive view of your operations. As you continue to put systems like this in place, your advanced analytics will become more and more valuable, helping you to improve forecasts, optimize transport and production networks, and ultimately reduce costs.
LATEST POSTS
- Understand Why Production Planning Needs Specialized Solutions
- Understand Circular Economy in The Manufacturing Industry
- How Can Industry 4.0 IT Integration Be Achieved Smoothly?
- The Significance of Order Sequencing in Discrete Manufacturing
- How to improve your Supply Chain Management: The Power of Control Towers