Forecast Accuracy: Demand Sensing in the Supply Chain
Brian Hoey - November 15, 2018
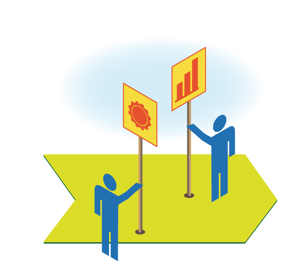
Let’s say, hypothetically, that you’re a big fan of baking, and every so often your officemates are happy to act as guinea pigs by trying out whatever inventive confections you dream up. The one problem here is that your business involves a lot of travel and a lot of semi-remote workers, so it can be difficult to estimate how many people are going to be in the office on a given day—i.e. it's tough to know how large a batch of cookies to bring in when you decide to bake for your coworkers. You might simply base your batch sizes on past demand levels, assuming that because the last time you baked there were X number of people in the office, similar numbers are likely to hold true again, but this strategy has the potential to miss the mark drastically.
On the other hand, you could sit down before you preheat your oven and look at your calendar. Are there any conferences this week that would keep people out of the office? Is there going to be inclement weather that might cause more people to work from home than usual? In this way, you could arrive at a better-informed number, and, with any luck, fewer of your baked goods would go to waste. If you were to choose this latter strategy, you’d actually be engaging in some rudimentary demand sensing. But what does this process look like in the context of the supply chain, and why are so many businesses adopting it to improve their forecasting accuracy?
The Trouble with Traditional Forecasts
You may be getting the feeling that demand sensing wasn’t really conceived as a tool for office bakers. Rather, it was developed as a response to the historical problems of forecasting demand. For more than a century, time series techniques were used to turn historical order and demand data into new demand forecasts, which businesses could then use to help guide their production workflows and inventory usage for the coming months or years. The issue with this state of affairs was (and is) that these kinds of estimates are often wildly inaccurate, frequently missing the mark by more than 50% in either direction. For years, this technique persisted because there were no especially viable alternatives. As the era of Big Data and the Fourth Industrial Revolution began to draw near, however, an increase in the amount of supply chain data being gathered and stored paved the way for a new approach, meaning that manufacturers could gain the opportunity to break out of these past-oriented forecasting cycles and thereby reduce needless inventory costs and expensive production overages.
Enter Demand Sensing
Unlike the forecasting techniques of the past, demand sensing can respond to a variety of factors that influence demand, from market downturns and raw material pricing shifts to weather patterns and inventory data, frequently running machine learning algorithms on these factors in order to determine their likely effects. In this way, forecasting error can be reduced, and huge swaths of the supply stream can be smoothed out. If your manufacturing outfit, for instance, is able to predict in advance that a certain part that you produce is going to see a decrease in demand this month (maybe because the automotive manufacturer who’s been utilizing it is pushing back its new model release for the year due to tariff concerns), you can adjust your production flow accordingly. By producing fewer of these parts, you avoid the possibility of being stuck with a warehouse full of unsold stock, thus saving money on storage costs. Or, to take another example, you might be in a pattern where the same client makes the same parts order at the same time every year. Instead of just assuming that this year will be the same, you rely on your demand sensing workflow, which notices that your client’s business is in the midst of a huge boom, and that due to changing weather patterns, seasonal orders have been creeping up slowly on the consumer end for a few years. Instead of being blindsided by an order that’s both larger and earlier than usual, you can ramp up production of the necessary parts to meet the impending order.
Implementation Challenges
Now, I know what you’re thinking: “if this is so effective, why isn’t everyone utilizing it?” Though demand sensing can be a game changer for supply chain management, there are operational hurdles that need to be overcome before it can be implemented successfully. Most importantly, demand sensing requires real-time supply chain data in order to thrive. This can be a difficult thing to achieve for some businesses, especially those that may be working with legacy IT systems or suffering from data silos. Industry 4.0 technologies like internet of things (IoT) devices and smart sensors on factory floors are generating more data than ever before, but in order to take advantage of them businesses need to make visibility and interoperability a priority. In this way, demand sensing workflows will have access to the internal data that they need, which can then be combined with information from up and downstream sources in the value chain to create smarter predictions.
This is, of course, easier said than done. But by undertaking initiatives to root out Shadow IT (i.e. software solutions that lack institutional buy-in and thus don’t integrate with other segments of your company’s IT infrastructure) and adopting a Postmodern ERP mindset (in order to link together disparate IT solutions into a cohesive, connected whole), it’s possible to meet the data requirements that these processes demand. In doing so, you can pave the way for a more accurate, more sophisticated method of forecasting demand—a method that can have a ripple effect on your production planning, inventory management, and transport logistics, resulting in increased efficiency and decreased waste.
LATEST POSTS
- Understand Why Production Planning Needs Specialized Solutions
- Understand Circular Economy in The Manufacturing Industry
- How Can Industry 4.0 IT Integration Be Achieved Smoothly?
- The Significance of Order Sequencing in Discrete Manufacturing
- How to improve your Supply Chain Management: The Power of Control Towers