Boosting Efficiency in Variant-Rich Manufacturing
Brian Hoey - January 05, 2021
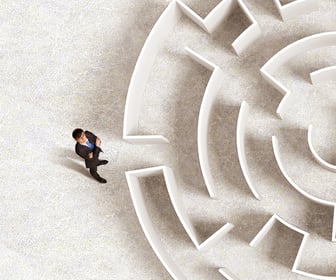
In a truly variant-rich production environment—like that of an automotive manufacturing plant—the possible paths for parts to take through the various workstations can quickly become too large to grapple with. As you layer in time and order constraints, that number shrinks, but it’s still too large for a human planner to deal with comprehensively. In the past, this meant that inefficiencies in the production process, especially in areas where there might be some degree of order customization within the main sequence, were more or less a given.
Now that digitization is a meaningful reality in the industrial sphere, those inefficiencies are finally getting easier to find and root out. Digital planning technology makes it possible to model your entire factory in virtual space in order to establish a more cohesive planning and scheduling process, which means that you can utilize AI and advanced analytics to go beyond the capabilities of any human planner when it comes to identifying more efficient schedules and sequences. As product customization becomes increasingly important to consumers and the rise of electric and autonomous vehicles disrupts the established automotive value chain, your ability to boost efficiency in this way will quickly become non-negotiable. The question is: where do you start?
Smarter Production Scheduling and Sequencing
First things first, in order to boost efficiency you need to optimize your sequencing and scheduling flows. Right now, each production run likely has a ton of variables and parameters—including complex time constraints, throughput times, workstation availabilities, resource needs and availabilities, etc.—and each individual production line might even have its own unique set of complex variables and concerns. Simply put, you’ll need a planning solution that can account for that complexity. Here, an AI-equipped APS system and related planning modules can be a huge boon. Why? Because they can automatically perform order slotting, sequencing, and scheduling passes to create smoothed, stable production runs based on emerging orders.
Rather than operating as a black box that spits out production sequences based on mysterious, inscrutable calculations, the ideal solution in this area will actually serve to increase your production visibility. It should help you to visualize your existing parameters and offer an interactive system by which you can modify existing plans to better match your production runs to your actual customer orders, logistics constraints, etc. This is what’s known as “explainable AI” or “user-centered AI,” and it operates on the principle that analytics processes can and should act as complements to—rather than replacements for—human planning. In this way, your technology becomes a way to cut through existing complexity, rather than a source of additional complexity. As a result, you’re able to successfully cut waste out of your production sequences and schedules.
Integrated Planning and Replanning
Increasing the efficiency of your actual production runs is a huge step towards cutting out waste from your manufacturing enterprise more broadly—but improvements to sequencing and slotting are more or less confined to the factory floor. This means that if you find yourself in a position where you have a surge in unexpected demand (unexpected, at least, from the perspective of the production planner), there’s a limit to how effectively you can plan and replan your way out of the situation. For this reason, true efficiency in a complex manufacturing environment comes from integration with other touchpoints up and down the value chain.
By way of example, let’s think about a company that has a robust S&OP process, where data-driven demand forecasts are used as the basis for annual capacity plans. The forecasts for the next several months all predict increased demand, which means that S&OP planners and other stakeholders will need to find a way to increase capacity—which will mean working production planners to understand and built out plant capacity, inventory planners to get a sense of how quickly you’ll burn through your buffer stock, and any logistics providers who might need to reserve capacity for your increased volume of product.
In this scenario, from the perspective of the factory floor operations themselves, the longer you wait to gain critical information from these other touchpoints, the less efficient your production flows will be. If a demand spike for a particular product is going to impact your production ratios for the next month, you want to know that sufficiently early on that you can implement optimized sequences and schedules from the very beginning. Unfortunately, many businesses up and down the supply chain don’t have the necessary technology integration to make this happen. As a result, they wind up in a position where production plants are scrambling to leverage their entire stock and capacity to meet demand, ultimately leading to significant use of premium freight to get products to customers, expensive rush restock orders, and machine wear and tear that can prove costly in the long run.
The Power of Proactive Machine Maintenance
Boosting efficiency in manufacturing via increased integration might seem a little bit abstract. To be sure, it’s an effective way to cut down on waste—especially in cases where you might be experiencing fluctuations in the market—but it’s hard to disentangle the efficiency gains from increased integration with the other benefits of a more connected and dynamic technology stack in your supply chain. Using predictive analytics to schedule proactive machine downtime and thus avoid breakdowns down the road, on the other hand, is a direct, straightforward way that even an incredibly complex production program can boost its efficiency through digital technology.
The basic premise here is fairly simple: machine learning algorithms trained on historical machine data establish correlations between different machine behaviors and the likelihood of an unexpected breakdown. When the signs of a looming breakdown become present, your ML-powered solution prompts to schedule some downtime in advance to perform maintenance on the machine. Since you’re not blindsided by an unexpected breakdown, you’re able to schedule the maintenance on your own terms, meaning that you can choose the time that’s least disruptive based on your current set of parameters. Thus, you avoid all of the wasted time that comes from unplanned breakdowns and you get more life out of your equipment. The result is that your production plant has less idle time, and is thus able to make more efficient use of its resources.
Learn More About Sequencing Here:
LATEST POSTS
- Understand Circular Economy in The Manufacturing Industry
- How Can Industry 4.0 IT Integration Be Achieved Smoothly?
- The Significance of Order Sequencing in Discrete Manufacturing
- How to improve your Supply Chain Management: The Power of Control Towers
- Optimizing Human Resource Scheduling in Manufacturing: A Technological Approach