Are Your Demand Forecasts Data-driven?
Brian Hoey - January 15, 2019
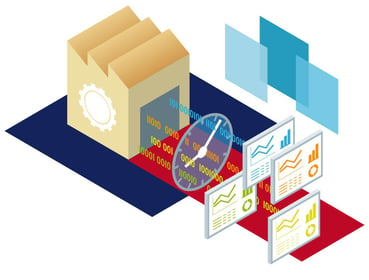
Let’s take a second to compare two hypothetical World Cup forecasts. Both forecasts are trying to determine who the likely winner of the contest will be, but their methods differ fairly radically. The first forecast starts out with team rosters, facts and figures, and all manner of statistics pertaining to the various players and teams. Based on those facts and figures, a statistician begins to derive and weight a set of probable outcomes. Those outcomes are sent on to a human prognosticator (an expert in the sport, perhaps a former player or coach or a newspaper commentator) who uses his experience and judgment to tweak the probabilities handed down to him by the statistician. The stats think that a particular player on the French team will age poorly, but the prognosticator thinks otherwise, and changes the predictions accordingly. After this first round of edits, the predictions are passed on to the next editor, who brings her own experience to bear, changing the projected outcomes yet again.
In the alternative forecast, all of the relevant data from that year and previous years is fed into a machine learning algorithm. The algorithm simulates the World Cup matchups continuously, and spits out the most likely outcomes based on those simulations. Which of these two forecasting models seems like it will better predict the outcome of the tournament? That’s right, it’s the one that doesn’t feature several rounds of human intervention where the biases and gaps in knowledge of the individual planners can skew the results. Why? Because the second forecast is data-driven.
What is Data-driven Forecasting?
At this point you might be wondering what, exactly, counts as a data-driven forecast. Basically, any forecast that primarily leverages data, rather than human knowledge or intuition, is said to be data-driven. This concept can be applied not just to sports prognostication, but to a manufacturing and industrial context as well. In the pre-Industry 4.0 era, most forecasts were able to leverage data only in a fairly limited way, referring to past customer and market behavior in order to pin down initial assumptions that could then be refined by human planners. This paradigm has its uses, but it’s fundamentally reactive, responding slowly or not at all to emerging changes in a given market and relying instead on the assumption that the past will always be instructive.
Data-driven forecasts, by contrast, often incorporate real-time data flows in order to create dynamic demand, market, and pricing predictions that utilize the complex interrelations between various and complex factors. That said, these forecasts are not solely based on external data streams. On the contrary, they make significant use of the operational data that is increasingly being collected in digitized manufacturing and shipping workflows. This can include inventory data, machine usage information, relative volumes and customer requirements, or any other information that's being made available. The more data available, generally speaking, the more accurate and thus more valuable the forecast.
Benefits of Being Data-driven
Okay, so we’ve seen the difference between these two modes of forecasting illustrated fairly clearly, but, what, exactly are the benefits of the demand driven version from a manufacturing perspective? As we alluded to above, the primary source of added value here is increased forecast accuracy. By removing much of the guesswork that comes with planner-intuition and unshackling forecasts from the assumption that the past is the only reliable predictor of the future, data-driven forecasts pave the way for significant improvements in accuracy—which in turn pave the way for all of the operational benefits associated with type of forecasting. Imagine, for instance, that rather than picking the winner of the World Cup, you’re trying to determine the right raw materials to source for your production workflows. Your two top selling products have substantially different bills of materials, so you need to have a firm idea of what your production ratio will be before you source your materials. Based on current demand levels, minute differences in material prices over previous years, trends in buyer behavior, and granular data on your customers and their buying habits, your advanced predictive analytics workflows create a forecast that comes much closer to the eventual demand reality than you would have been likely to get with a manual process. This means that you source your raw materials in the right ratios for your upcoming production program, and as a result you don’t find yourself facing large storage costs for materials that will go unused. Likewise, because your production workflows represent reality more closely, fewer of your finished products wind up occupying costly warehouse space. In this way, costs are kept down and your supply chain is kept running smoothly.
Achieving Data Integration
At this point you might be asking your, “If data-driven forecasts are so much better, why doesn’t everyone use them?” After all, the benefits outlined above can make a big difference to a company’s bottom line. Though these workflows can be impactful, they also have high requirements for data integration in order to be implemented successfully. What does this mean? Essentially that your entire supply chain, from suppliers to production workflows to transportation logistics, have to be positioned to collect data, either through IoT devices, RFID chips, or other digitized means. Not only must this data be collected, it must be stored in an accessible location where planners and analytics workflows can access them with a minimum of fuss. This might not sound too difficult, but many organizations will have a lack of cohesive IT integration and a proliferation of data silos that makes this level of data quality and accessibility hard to come by.
To optimize your value chain for better forecasts, start by adopting an IT infrastructure that privileges visibility, ideally centralizing data mission critical data into one database. With that infrastructure in place, you can begin to introduce multiple information streams into your analytics workflows, creating an environment in which they can provide you with more accurate predictions. In this way, you can smooth out your operations and avoid the supply chain disruptions that come with poor demand planning—from expensive, unwanted stock overages to late customer deliveries.
LATEST POSTS
- Understand Why Production Planning Needs Specialized Solutions
- Understand Circular Economy in The Manufacturing Industry
- How Can Industry 4.0 IT Integration Be Achieved Smoothly?
- The Significance of Order Sequencing in Discrete Manufacturing
- How to improve your Supply Chain Management: The Power of Control Towers