Advanced Supply Chain Analytics 101
Kristin Masters - November 10, 2016
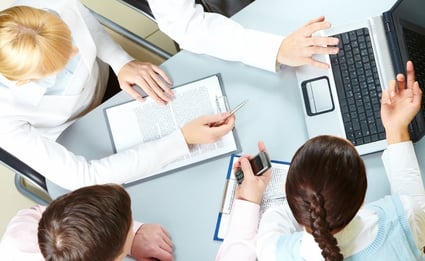
Supply chain leaders have long incorporated data into their decision-making processes, and analytics have played a vital role in raising supply chain visibility. As we enter the era of Industry 4.0. supply chain visibility will become more important than ever; a more complex supply chain with more distributed suppliers and customers demands equally robust analytics.
Supply chain leaders have long incorporated data into their decision-making processes, and analytics have played a vital role in raising supply chain visibility. As we enter the era of Industry 4.0. supply chain visibility will become more important than ever; a more complex supply chain with more distributed suppliers and customers demands equally robust analytics.
Thus the manufacturing industry has a shared vision for migrating to an integrated system of advanced analytics, where systems are able to offer actionable information and recommendations, rather than merely an analysis of what has already happened. The first step toward making that vision a reality is understanding the scope and applications of advanced analytics.
What Are Advanced Analytics?
Most supply chain managers rely on analytics daily. The analytics deployed in most supply chains fall into these two categories:
- Descriptive analytics are used for supply chain performance management dashboards and reports, along with event management. Examples of descriptive analytics include visualization, clustering, association and reporting.
- Diagnostic analytics are useful for root cause analysis (RCA), online analytical processing (OLAP), and what-if analytics. They are commonly applied for RCA of supply chain performance and data visualization.
Both descriptive and diagnostic analytics are passive, offering insight only into what has already happened. While these analytics are indispensable to the supply chain, they are not advanced because the end user must still imagine future scenarios and make strategic predictions.
Advanced analytics, on the other hand, provide that information about the future. They fall into two general categories:
- Predictive analytics assist with activities like supplier risk management, demand forecasting and network simulation. Predictive analytics include machine learning and causal forecasting, in addition to simulation and scenario management.
- Prescriptive analytics allows optimization of transportation routes, factory scheduling and inventory. Stochastic and deterministic optimization are both considered prescriptive analytics functions, as is rapid replanning optimization.
Benefits of Advanced Analytics
Both diagnostic and descriptive analytics offer key insights into supply chain operation, providing necessary data for critical decision making. But they are limited in scope, and it can also be difficult to track their ROI. This is why many manufacturing organizations don't push their data capacity further; it's difficult to justify investment in advanced analytics when the current analytics infrastructure yields only nebulous impact on the bottom line. But an investment in advanced analytics results in multiple measurable benefits:
- Improved asset utilization, product quality and customer service
- Lower inventory costs
- Shorter order cycle time
- More successful product launches
- Higher revenue
It's no wonder, then, that in a recent Gartner survey, respondents with the highest analytics maturity also reported the highest ROI. Advanced analytics build on one another, creating a sort of feedback loop that can optimize every step in the supply chain.
Advanced Analytics, Machine Learning and Human Judgment
In addition to understanding the definition of advanced analytics, supply chain managers must also understand the definition and applications of machine learning. While machine learning can provide predictive and prescriptive analytics, it is not the only mechanism for acquiring them. Simply put, machine learning is an analytics technology that can teach itself to predict and make decisions based on analysis; it can analyze past performance in the context of new data to continually refine its activities.
Even in the age of machine learning and advanced analytics, however, human judgment still plays a critical role in supply chain management. Human judgment is still necessary for a number of functions:
- Conceiving, creating and training machines
- Making decisions in complicated environments where there is no one correct answer
- Refining data and analytics output in response to new information or circumstances
When paired with both human judgment and machine learning, advanced analytics are incredibly powerful. It's important never to overlook the role of human resources in the implementation and deployment of advanced analytics.
Successfully Adopting Advanced Analytics
Adopting any new capability or technology requires planning, and advanced analytics are no different. Advanced analytics must build on the supply chain's existing data framework, in cooperation with human operators. To that end, there are four key steps to successfully implementing advanced analytics for supply chain management:
- Identify the most critical use cases. Clearly define exactly which advanced analytics applications will be most immediately valuable. For most supply chain leaders, the most critical use cases will fall into the category of predictive analytics, which build on their current diagnostic and descriptive analytics.
- Determine required data sources. Does your supply chain already have the ability to provide the required advanced analytics? Diagnose and address any gaps before layering on new technologies and techniques.
- Recognize necessary human resources. People must install, operate and interact with even the most sophisticated advanced analytics systems. Ensure that you have sufficient manpower for the implementation of a more complex system.
- Select the appropriate advanced analytics technologies and techniques. Choosing the right methodology and technology will smooth the transition to a more robust analytics system.
Ultimately the widespread adoption of analytics will drive the manufacturing industry into a new era of interconnectedness and distribution. Supply chain leaders who embrace these techniques and technologies will be poised to lead their organiations into the future.
If you want to learn more, download your guide to Transformation of Manufacturing Processes.
In this Guide you will learn:
-
Emerging Challenges in the Modern Truck/Automotive Industry
-
How Can Global Companies Adapt to These New Realities
-
How Decentralized Digital Systems Power Smarter Planning Processes
-
How flexis Can Support Flexible Supply Chain Transformation
LATEST POSTS
- Understand Why Production Planning Needs Specialized Solutions
- Understand Circular Economy in The Manufacturing Industry
- How Can Industry 4.0 IT Integration Be Achieved Smoothly?
- The Significance of Order Sequencing in Discrete Manufacturing
- How to improve your Supply Chain Management: The Power of Control Towers