What Is Job Shop Scheduling?
Brian Hoey - February 05, 2019
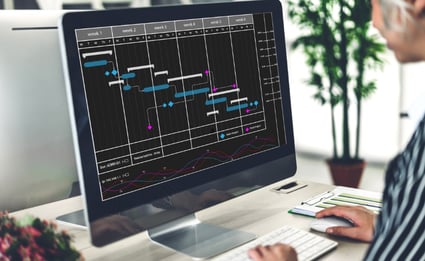
Historically, we tend to think of Henry Ford’s adoption of the assembly line as one of the most important moments in the history of automotive manufacturing—a moment when, all of a sudden, automobiles could be produced in an efficient, cost effective way. While it’s certainly true that this was a watershed moment for the industry, this emphasis on the assembly line can have the effect of obscuring that other production scheme that’s so integral to lives of many modern auto makers: the job shop. Indeed, job shop environments are crucial in many production workflows for the creation of parts and even whole cars. As a result, the ability to create efficient production schedules in such an environment can be a key value added propositions for manufacturers.
Mapping the Job Shop
In order to really understand the challenges and rewards that come with effective job shop scheduling, we first have to outline what, exactly, constitutes a job shop. Unlike an assembly line, which may feature a number of machines that are always used in succession, a job shop will typically feature a set of machines that can be used in multiple different orders and combinations depending on what part or product is being produced. As a result, these production workflows are typically non-timed or non-clocked (as opposed to timed production workflows in which each step can be quickly mapped onto a reproducible timetable), meaning that they don’t have an inherent schedule intrinsic to their designs or layouts.
In an automotive context this non-linear configuration of machines might take many forms. A single machine or group of machines might be used for applying paint to both car doors and other, smaller parts, even though their respective workflows up to that point don’t feature many overlapping machines or processes. By the same token, you might use the same set of machines in a different order to create different elements of the same engine. The variety of uses for each machine helps businesses to maintain flexibility on their production floor, but it also creates potential optimization issues. Imagine for a second that, as we described above, you’re using the same machine for painting multiple different parts: these parts are created via different processes that have different timetables, and as a result the optimal way for the two parts to share the machine’s time is not obvious. You want to minimize the makespan of each and reduce machine downtime, but your production ratios may require you to sacrifice some of your uptime to the realities of each parts’ respective timelines. This, in a nutshell, is where job shop scheduling becomes such a critical piece of the production planning puzzle.
Solving the Job Shop Problem
As it happens, the job shop problem isn’t just a sometimes-inconvenient fact of life for manufacturers. It’s also an important question in math and computer science—and it’s not hard to see why. If, as we’ve seen above, you have a set of potentially complex jobs that must be run on a limited number of machines in a very particular sequence, the odds of a human planner finding the optimal path for each product by hand are slim. Even for computer scientists, there is no one size fits all solution for scheduling tasks in a job shop. Thus, like the slightly better known traveling salesman problem, the job shop problem is considered to be NP-Hard, meaning that no one has yet discovered an algorithm that produces the optimal schedule (i.e. the schedule that completes the desired set of jobs in the shortest amount of time) in every single instance. As a result, there’s a proliferation of different methods for maximizing job shop efficiency.
What is the practical upshot of all these theoretical investigations into a very real manufacturing phenomenon? Essentially, once we’ve identified job shop scheduling as a complex problem, we can begin to undertake more sophisticated solutions. A production planner who’s handed a schematic of a job shop and a given a demand snapshot for the coming year might be able to cobble something together for planning purposes, but the creation of each production sequence is going to involve to many variables and too many moving parts for optimization by hand—and this is before we factor in disruptions and future changes to production requirements and demand levels. Though much of the value of the job shop environment comes from its inherent flexibility, the wide variety of potential paths each product can take through the production environment actually makes it more difficult for manual planning processes to happen on the fly, meaning that whenever reality diverges from your expectations it will be hard to preserve value.
Theory Meets Practice
Like we suggested above, a complex problem will often require a complex solution. Thus, job shop scheduling is, in general, a process best undertaken in a smart, digital environment. In this way, it has dovetailed nicely with the rise of Industry 4.0 and the connected smart factory. As auto manufacturers are beginning to create the kinds of cyber-physical systems that will define the Fourth Industrial Revolution, job shop scheduling processes are evolving to encompass new data and new possibilities. By mapping out their production environments into digital space (in a highly visible, legible, and data-rich way), businesses are becoming more equipped to leverage the theoretical work that's been done on this question over the years, creating automated scheduling and analytics workflows that leverage data to create usable plans
In an automated, digital environment, the complexity of job shop scheduling as a task can finally be addressed head on. As hurdles and disruptions arise, production programs can be replanned in order to preserve maximum value. Thus, where job shop scheduling was once a fairly siloized activity, it’s lately becoming more and more integrated into other Industry 4.0 processes. Demand capacity planning, inventory planning, and transport logistics workflows are increasingly able to communicate with production plans even in non-timed environments, which creates a smoothing effect across the entire value chain. Looking towards the future, we expect to see these planning workflows becoming increasingly responsive to demand shifts, increasingly flexible and adaptable, and less susceptible to machine breakdowns and errors within individual jobs. If traditional job shop scheduling represents a puzzle that needs solving, modern, digitized job shop production should look more like the entire digital supply chain in microcosm: a system where smart planners can count on finding smart ways to manage ever-shifting capacities and availabilities.
If you want to learn more get your Guide to Industry 4.0:
LATEST POSTS
- Understand Why Production Planning Needs Specialized Solutions
- Understand Circular Economy in The Manufacturing Industry
- How Can Industry 4.0 IT Integration Be Achieved Smoothly?
- The Significance of Order Sequencing in Discrete Manufacturing
- How to improve your Supply Chain Management: The Power of Control Towers